Data analytics is integral to modern business strategies, driving crucial decision-making processes. However, the journey from raw data to actionable insights is often riddled with challenges. Data quality, integration, interpretation and implementation often impede progress. They hide the true value of data. To master each of those pieces, you want to work your way up the data analytics hierarchy, ensuring data governance at each step.
The data analytics hierarchy — comprising data, descriptive, diagnostic, predictive, prescriptive and proactive analytics — is key to getting the most out of your data. When you start to layer in generative AI, you can really unlock the potential of your data.
Data analytics pain points
We all collect data, but do we collect data correctly? Are we collecting it so we can do something with it? Below are some problems caused by a lack of data governance.
Data overload
Collecting data is easy. Once we’ve had a piece of software, we just plug it in and let it run. What ends up happening is that companies then have a large pile of data to sort through. It’s akin to walking into a hoarding situation.
Data is everywhere, and it will take a long time and a lot of patience to organize it and extract what’s useful. Companies grapple with managing and retrieving relevant information from vast data volumes.
Data quality issues
With data overload comes inaccurate data. Because you’ve collected so much of it, it’s difficult to know what data to use. Companies have a harder time sorting the wheat from the chaff. Inaccurate or inconsistent data leads to flawed analysis and decision-making.
Integration challenges
Every vendor wants you to be loyal to them and their suite of software. In reality, most companies are using a variety of tools that collect and export different data sets, for different needs. Merging data from various sources is complex and time-consuming when data governance is lacking.
Delayed insights
With poor data governance, companies are stuck in reactive mode. They struggle to get ahead and are always waiting to find out what happened. This results in an inability to make timely decisions.
Businesses can improve their data-driven decision-making by addressing these challenges through the data analytics hierarchy. Let’s explore each level and the role of generative AI in enhancing each phase.
Dig deeper: Breaking down data silos: Overcoming obstacles and planning for the future
The data analytics hierarchy
The data analytics hierarchy is a structured approach. It ensures a full understanding and use of data. It consists of six levels, each building upon the previous one to provide deeper insights and more actionable outcomes:
- Data: The raw, unprocessed information collected from various sources.
- Descriptive analysis: Summarizes historical data to identify trends and patterns, answering “What happened?”
- Diagnostic analysis: Explores the underlying reasons behind observed trends, answering “Why did it happen?”
- Predictive analysis: Uses historical data to forecast future events, answering “When will it happen?”
- Prescriptive analysis: Recommends specific actions based on predictive insights, answering “What should we do about it?”
- Proactive analysis: Involves AI agents that autonomously execute recommended actions, answering “Can the machine do it for me?”
Each level in this hierarchy is crucial for effective data-driven decision-making. Let’s delve into each phase in detail and see how generative AI enhances each one.
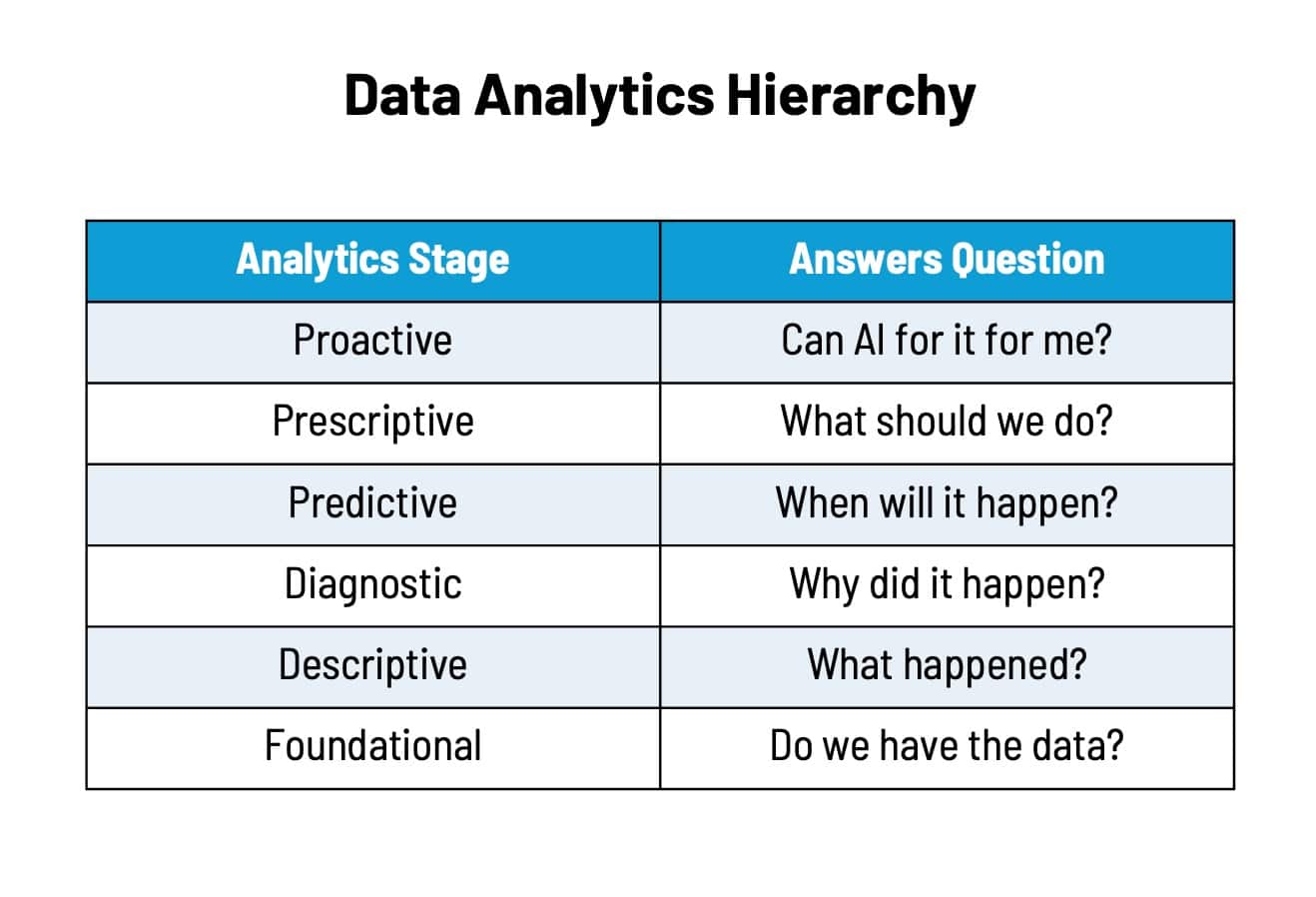
1. Data: The foundation
Specific pain point
- Managing the sheer volume and variety of collected data is overwhelming.
Solution
- Establishing a robust data foundation involves collecting, cleaning and storing data from various sources. Before you start any kind of data collection, you’ll want to put together requirements.
- Outline your business goals, KPIs and user stories. Knowing what data you want to collect and how you will use it will guide the setup of your systems.
Generative AI applications
- Synthetic data creation: Generative AI can produce synthetic data to supplement real-world data, ensuring diverse and robust training datasets.
- Data normalization: AI algorithms automate data normalization, ensuring consistency and accuracy across datasets.
2. Descriptive analysis: What happened?
Specific pain point
- Extracting meaningful insights from unstructured and voluminous data is a challenge.
Solution
- Descriptive analytics summarizes historical data to identify trends and patterns. This is typically quantitative data from your CRM, web analytics and marketing automation systems.
- When companies set up these systems, they tend to “set it and forget it” rather than spending time properly configuring them. By starting from the foundation, you know what data you should be collecting, how you’ll extract it and what insights you can glean.
Generative AI applications
- Code development: AI can assist you in writing code that can expedite data extraction and analysis.
- Automated data exploration: AI explores data relationships automatically, uncovering insights often missed through manual analysis.
- Data visualization: Generative AI creates attractive visualizations that highlight key insights and help with data understanding and communication.
3. Diagnostic analysis: Why did it happen?
Specific pain point
- Identifying the root causes of trends and anomalies.
- Many companies skip this step because data collection can be straightforward, but data analysis can be daunting. They’re left with a mound of unstructured qualitative data that is difficult to analyze.
Solution
- Diagnostic analytics seeks to understand the reasons behind observed trends.
- Once you understand what happened, the next logical step is to determine why it happened. This comes through customer feedback, market research and monitoring trends.
Generative AI applications
- Summarization: Generative AI can ingest all your qualitative data and extract patterns and trends. Companies can upload survey data, feedback questionnaires and even other market research and white papers. It can summarize the common points and assist in creating actionable plans based on the data.
4. Predictive analysis: When will it happen?
Specific pain point
- Accurately forecasting future trends in dynamic environments.
- As marketers, we’ve tended to rely on instinct and anecdotal evidence to plan our campaigns and efforts.
Solution
- Predictive analytics uses historical data to forecast future events.
- Forecasting is a powerful, yet underutilized tool. It’s most effective when you have a strong foundation of qualitative and quantitative data with good data governance.
Generative AI applications
- Enhanced forecasting models: AI builds and refines predictive models, simulating various scenarios to provide a range of potential futures.
- Code generation for custom models: AI writes and optimizes code for complex predictive models, reducing development time and expertise requirements.
5. Prescriptive analysis: What should we do about it?
Specific pain point
- Determining actionable steps based on data analysis and insights is laborious.
Solution
- Prescriptive analytics recommends specific actions based on predictive insights. This is your plan, your direction.
- Gathering the data from the previous steps takes time. Marketers want to jump straight in and start taking action.
Generative AI applications
- Actionable recommendations: AI suggests detailed action plans by analyzing predictive insights and historical data, guiding the best course of action. You can ask for plans without using your data. However, nailing down the descriptive, diagnostic and predictive steps means you’ll be able to create highly tailored plans.
6. Proactive analysis: Can the machine do it for me?
Specific pain point
- It’s a challenge to quickly and effectively implement insights. Marketers are pulled in so many directions and if they only had some help, they could accomplish more.
Solution
- Proactive analytics involves AI agents autonomously executing recommended actions.
- Your data governance needs to be tight and accurate to reach this step. AI is acting and executing on your behalf, so it’s essential that you give the systems the right data.
Generative AI applications
- Autonomous decision-making: AI powers systems that make and act on decisions in real time, such as adjusting marketing strategies autonomously.
- Continuous learning and adaptation: AI agents continuously learn from new data, improving their performance and adapting to changing conditions without human intervention.
Dig deeper: How to make sure your data is AI-ready
Accelerating data-to-insights with generative AI
Generative AI is a transformative tool that enhances each step of the data analytics hierarchy. From creating synthetic data to generating actionable recommendations and autonomous decision-making, AI addresses common data analytics pain points.
By integrating generative AI into your processes, your business can achieve new levels of efficiency, accuracy and intelligence, transforming data into a powerful asset that drives success.
Email:
See terms.
The post The data analytics hierarchy: Where generative AI fits in appeared first on MarTech.